Querying MongoDB Data with BI Connector as a Graph
Summary
In this tutorial, you will:
- Create a Local MongoDB database with BI connector and load it with example data;
- Start a PuppyGraph Docker container and query the MongoDB data as a graph.
Prerequisites
Please ensure that docker compose
is available. The installation can be verified by running:
See https://docs.docker.com/compose/install/ for Docker Compose installation instructions and https://www.docker.com/get-started/ for more details on Docker.
Accessing the PuppyGraph Web UI requires a browser. However, the tutorial offers alternative instructions for those who wish to exclusively use the CLI.
Deployment
Create a file
docker-compose.yaml
with the following content:
docker-compose.yaml
networks:
puppy_net:
name: puppy-mongo
services:
hive-hdfs:
image: mongodb/mongodb-community-server:latest
container_name: mongodb
networks:
puppy_net:
ports:
- "3307:3307"
puppygraph:
image: puppygraph/puppygraph:stable
container_name: puppygraph
environment:
- PUPPYGRAPH_USERNAME=puppygraph
- PUPPYGRAPH_PASSWORD=puppygraph123
networks:
puppy_net:
ports:
- "7687:7687"
- "8081:8081"
- "8182:8182"
Warning: Ensure to modify your password environment variables, particularly when your machine is publicly accessible.
Then run the following command to start MongoDB and PuppyGraph:
[+] Running 3/3
✔ Network puppy-mongo Created 0.1s
✔ Container mongodb Started 3.7s
✔ Container puppygraph Started 3.7s
Data Preparation
This tutorial is designed to be comprehensive and standalone, so it includes steps to populate data in MongoDB. In practical scenarios, PuppyGraph can query data directly from existing tables in your MongoDB.
Run the following command to start a MongDB shell to access database:
This command will default access the modern
database like follow:
Current Mongosh Log ID: 679363652b2703e9db544ca6
Connecting to: mongodb://127.0.0.1:27017/modern?directConnection=true&serverSelectionTimeoutMS=2000&appName=mongosh+2.3.8
Using MongoDB: 7.0.16
Using Mongosh: 2.3.8
For mongosh info see: https://www.mongodb.com/docs/mongodb-shell/
To help improve our products, anonymous usage data is collected and sent to MongoDB periodically (https://www.mongodb.com/legal/privacy-policy).
You can opt-out by running the disableTelemetry() command.
------
The server generated these startup warnings when booting
2025-01-24T09:54:37.638+00:00: Using the XFS filesystem is strongly recommended with the WiredTiger storage engine. See http://dochub.mongodb.org/core/prodnotes-filesystem
2025-01-24T09:54:38.355+00:00: Access control is not enabled for the database. Read and write access to data and configuration is unrestricted
2025-01-24T09:54:38.355+00:00: vm.max_map_count is too low
------
modern>
Create the demo graph. We strongly recommend user to specify JSON schema validation to avoid errors.
db.createCollection("person", {
validator: {
$jsonSchema: {
bsonType: "object",
required: [ "id", "name", "age" ],
properties: {
id: { bsonType: "string" },
name: { bsonType: "string" },
age: { bsonType: "int"}
}
}
}
})
db.person.insertMany([
{id: 'v1', name: 'marko', age: 29},
{id: 'v2', name: 'vadas', age: 27},
{id: 'v4', name: 'josh', age: 32},
{id: 'v6', name: 'peter', age: 35}
])
db.createCollection("software", {
validator: {
$jsonSchema: {
bsonType: "object",
required: [ "id", "name", "lang" ],
properties: {
id: { bsonType: "string" },
name: { bsonType: "string" },
lang: { bsonType: "string" }
}
}
}
})
db.software.insertMany([
{id: 'v3', name: 'lop', lang: 'java'},
{id: 'v5', name: 'ripple', lang: 'java'}
])
db.createCollection("created", {
validator: {
$jsonSchema: {
bsonType: "object",
required: [ "id", "from_id", "to_id", "weight" ],
properties: {
id: { bsonType: "string" },
from_id: { bsonType: "string" },
to_id: { bsonType: "string" },
weight: { bsonType: "double" }
}
}
}
})
db.created.insertMany([
{id: 'e9', from_id: 'v1', to_id: 'v3', weight: 0.4},
{id: 'e10', from_id: 'v4', to_id: 'v5', weight: Double(1.1)},
{id: 'e11', from_id: 'v4', to_id: 'v3', weight: 0.4},
{id: 'e12', from_id: 'v6', to_id: 'v3', weight: 0.2}
])
db.createCollection("knows", {
validator: {
$jsonSchema: {
bsonType: "object",
required: [ "id", "from_id", "to_id", "weight" ],
properties: {
id: { bsonType: "string" },
from_id: { bsonType: "string" },
to_id: { bsonType: "string" },
weight: { bsonType: "double" }
}
}
}
})
db.knows.insertMany([
{id: 'e7', from_id: 'v1', to_id: 'v2', weight: 0.5},
{id: 'e8', from_id: 'v1', to_id: 'v4', weight: Double(1.1)}
])
The above SQL creates the following tables under the modern
schema:
id | name | age |
---|---|---|
v1 | marko | 29 |
v2 | vadas | 27 |
v4 | josh | 32 |
v6 | peter | 35 |
id | name | lang |
---|---|---|
v3 | lop | java |
v5 | ripple | java |
id | from_id | to_id | weight |
---|---|---|---|
e7 | v1 | v2 | 0.5 |
e8 | v1 | v4 | 1.1 |
id | from_id | to_id | weight |
---|---|---|---|
e9 | v1 | v3 | 0.4 |
e10 | v4 | v5 | 1.1 |
e11 | v4 | v3 | 0.4 |
e12 | v6 | v3 | 0.2 |
Install and Start BI Connector inside MongoDB container
BI Connector provides a relational schema and translates SQL queries between your BI tool and MongoDB. BI Connector is not default installed in the docker container. We need to dowload from website and install it inside the MongoDB container.
Enter into the
mongodb
container:
Confirm the OS system of the container:
DISTRIB_ID=Ubuntu
DISTRIB_RELEASE=22.04
DISTRIB_CODENAME=jammy
DISTRIB_DESCRIPTION="Ubuntu 22.04.5 LTS"
Download BI Connector according the OS system and your machine platform:
In x86_64 platform
wget https://info-mongodb-com.s3.amazonaws.com/mongodb-bi/v2/mongodb-bi-linux-x86_64-ubuntu2204-v2.14.21.tgz
wget https://info-mongodb-com.s3.amazonaws.com/mongodb-bi/v2/mongodb-bi-linux-x86_64-ubuntu2204-v2.14.21.tgz.md5
md5sum -c mongodb-bi-linux-x86_64-ubuntu2204-v2.14.21.tgz.md5
tar -zxvf mongodb-bi-linux-x86_64-ubuntu2204-v2.14.21.tgz
cd mongodb-bi-linux-x86_64-ubuntu2204-v2.14.21
In arm64 platform
wget https://info-mongodb-com.s3.amazonaws.com/mongodb-bi/v2/mongodb-bi-linux-arm64-ubuntu2204-v2.14.21.tgz
wget https://info-mongodb-com.s3.amazonaws.com/mongodb-bi/v2/mongodb-bi-linux-arm64-ubuntu2204-v2.14.21.tgz.md5
md5sum -c mongodb-bi-linux-arm64-ubuntu2204-v2.14.21.tgz.md5
tar -zxvf mongodb-bi-linux-arm64-ubuntu2204-v2.14.21.tgz
cd mongodb-bi-linux-arm64-ubuntu2204-v2.14.21
Start BI Connector.
Modeling a Graph
We then define a graph on top of the data tables we just created. Actually, this is the "Modern" graph defined by Apache Tinkerpop.
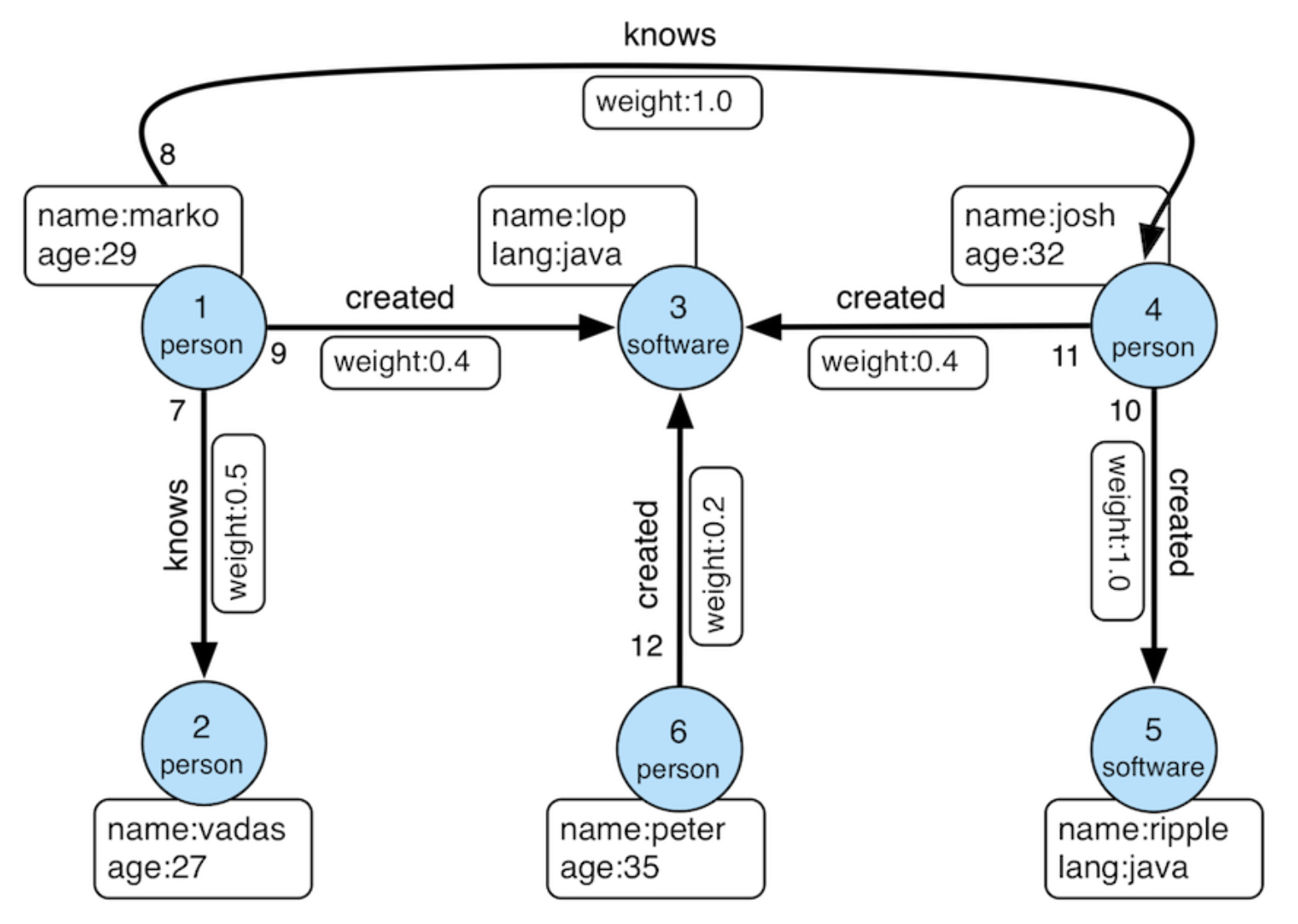
Modern Graph
A schema instructs PuppyGraph on mapping data from the Hive into a graph. PuppyGraph offers various methods for schema creation. For this tutorial, we've already prepared a schema to help save time.
Create a PuppyGraph schema file
schema.json
with the following content:
schema.json
{
"catalogs": [
{
"name": "mongodb_data",
"type": "mongodb",
"jdbc": {
"username": "",
"password": "",
"jdbcUri": "jdbc:mysql://mongodb:3307",
"driverClass": "org.mariadb.jdbc.Driver",
"driverUrl": "https://repo1.maven.org/maven2/org/mariadb/jdbc/mariadb-java-client/2.7.12/mariadb-java-client-2.7.12.jar"
}
}
],
"graph": {
"vertices": [
{
"label": "person",
"oneToOne": {
"tableSource": {
"catalog": "mongodb_data",
"schema": "modern",
"table": "person"
},
"id": {
"fields": [
{
"type": "String",
"field": "id",
"alias": "id"
}
]
},
"attributes": [
{
"type": "Long",
"field": "age",
"alias": "age"
},
{
"type": "String",
"field": "name",
"alias": "name"
}
]
}
},
{
"label": "software",
"oneToOne": {
"tableSource": {
"catalog": "mongodb_data",
"schema": "modern",
"table": "software"
},
"id": {
"fields": [
{
"type": "String",
"field": "id",
"alias": "id"
}
]
},
"attributes": [
{
"type": "String",
"field": "lang",
"alias": "lang"
},
{
"type": "String",
"field": "name",
"alias": "name"
}
]
}
}
],
"edges": [
{
"label": "knows",
"fromVertex": "person",
"toVertex": "person",
"tableSource": {
"catalog": "mongodb_data",
"schema": "modern",
"table": "knows"
},
"id": {
"fields": [
{
"type": "String",
"field": "id",
"alias": "id"
}
]
},
"fromId": {
"fields": [
{
"type": "String",
"field": "from_id",
"alias": "from_id"
}
]
},
"toId": {
"fields": [
{
"type": "String",
"field": "to_id",
"alias": "to_id"
}
]
},
"attributes": [
{
"type": "Double",
"field": "weight",
"alias": "weight"
}
]
},
{
"label": "created",
"fromVertex": "person",
"toVertex": "software",
"tableSource": {
"catalog": "mongodb_data",
"schema": "modern",
"table": "created"
},
"id": {
"fields": [
{
"type": "String",
"field": "id",
"alias": "id"
}
]
},
"fromId": {
"fields": [
{
"type": "String",
"field": "from_id",
"alias": "from_id"
}
]
},
"toId": {
"fields": [
{
"type": "String",
"field": "to_id",
"alias": "to_id"
}
]
},
"attributes": [
{
"type": "Double",
"field": "weight",
"alias": "weight"
}
]
}
]
}
}
Warning: Please use MySQL 5.7.X Community Edition (Or MariaDB 2.7.x )and the latest version of MongoDB Connector for BI. The plugin is not compatible with MySQL Server or Connector/ODBC driver version 8 and later.
Here we use MariaDB 2.7.12 Client to connect.
Log into PuppyGraph Web UI at http://localhost:8081 with username
puppygraph
and password puppygraph123
.
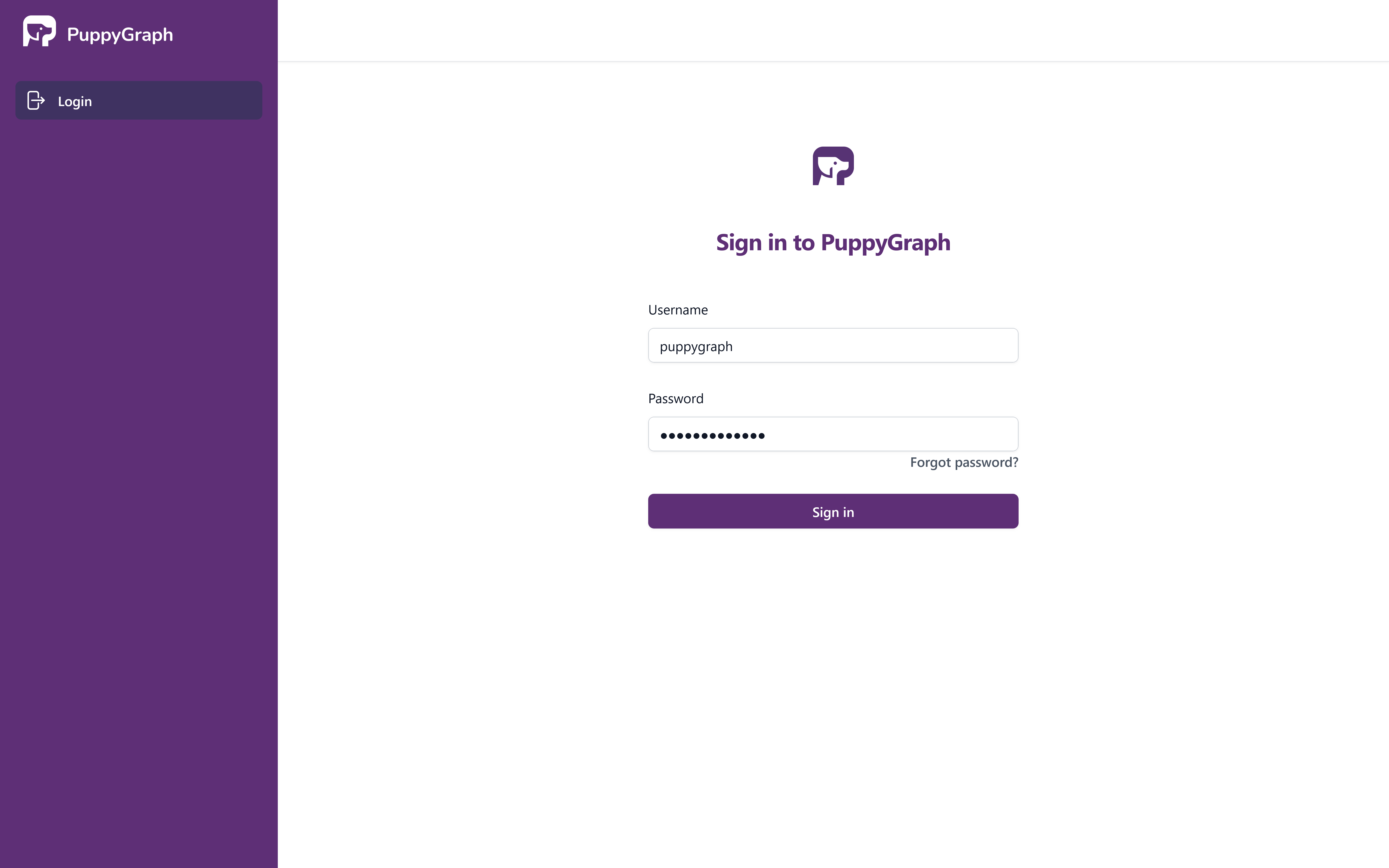
PuppyGraph Login
Upload the schema by selecting the file
schema.json
in the Upload Graph Schema JSON
block and clicking on Upload
.
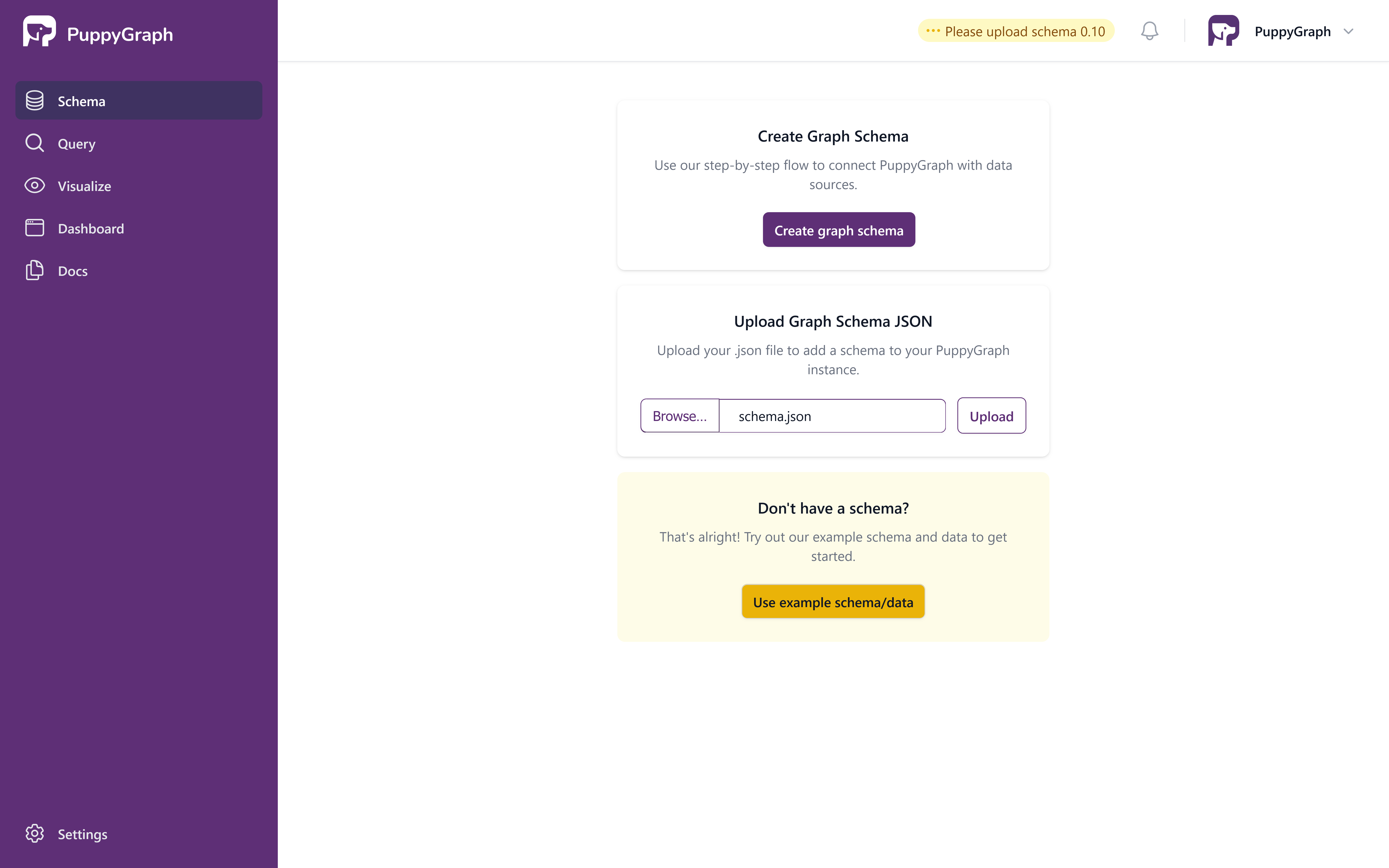
Upload Schema Page
Once the schema is uploaded, the schema page shows the visualized graph schema as follows.
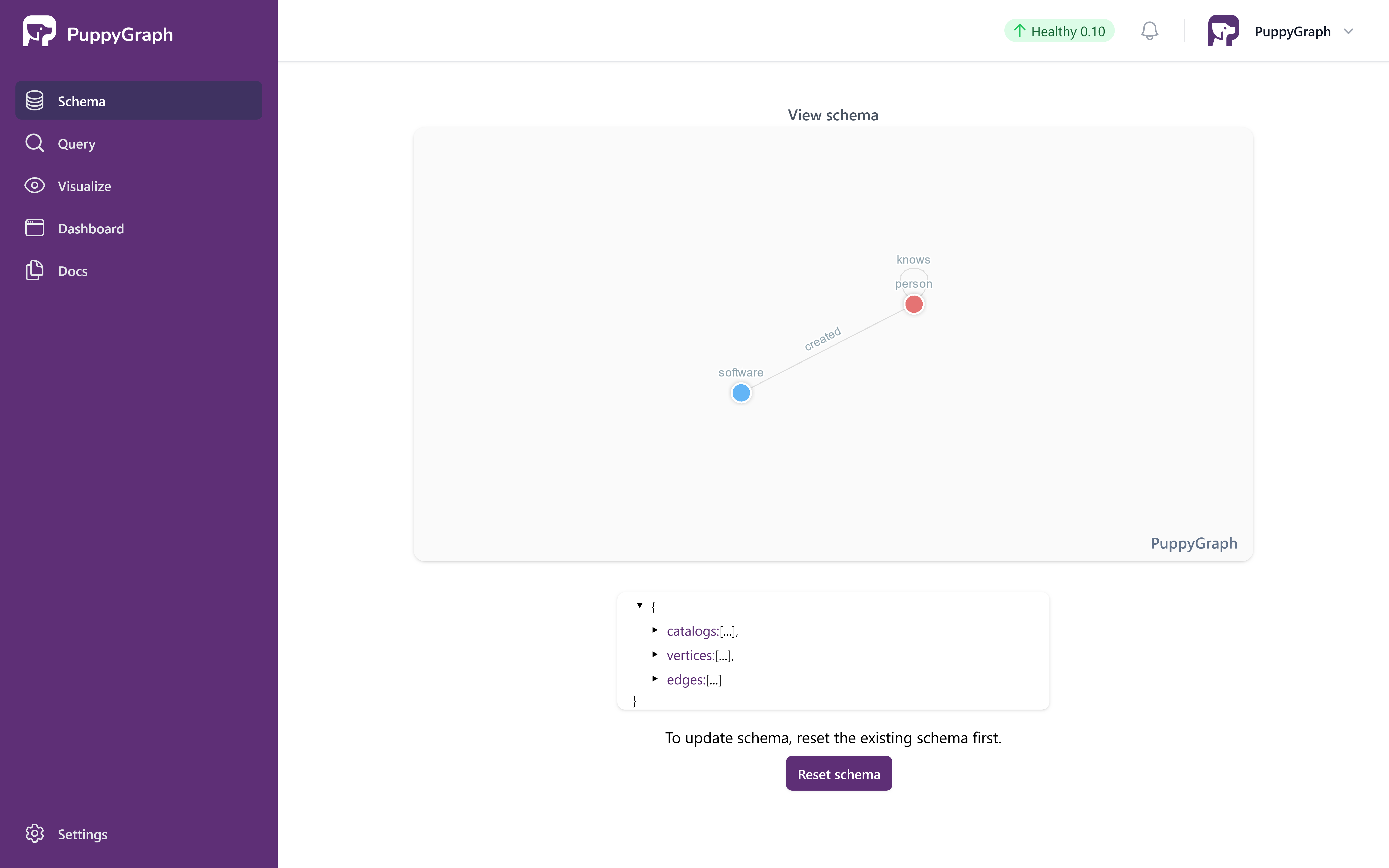
Visualized Schema
Alternative: Schema Uploading via CLI
Alternatively, run the following command to upload the schema file:
curl -XPOST -H "content-type: application/json" --data-binary @./schema.json --user "puppygraph:puppygraph123" localhost:8081/schema
The response shows that graph schema has been uploaded successfully:
Querying the Graph
In this tutorial we will use the Gremlin query language to query the Graph. Gremlin is a graph query language developed by Apache TinkerPop. Prior knowledge of Gremlin is not necessary to follow the tutorial. To learn more about it, visit https://tinkerpop.apache.org/gremlin.html.
Click on the
Query
panel the left side. The Gremlin Query tab offers an interactive environment for querying the graph using Gremlin.
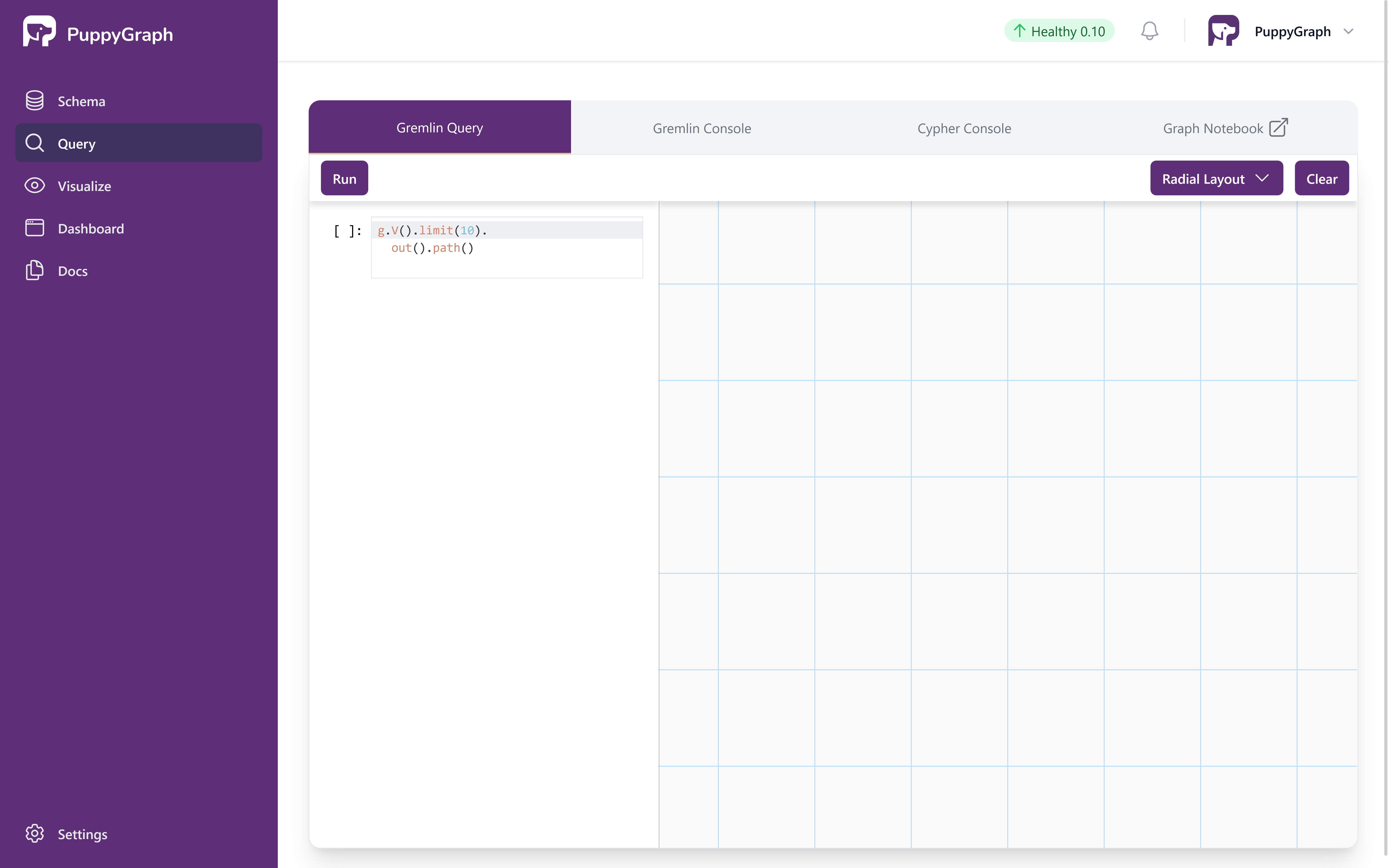
Interactive Gremlin Query Page
Queries are entered on the left side, and the right side displays the graph visualization.
The first query retrieves the property of the person named "marko".
Copy the following query, paste it in the query input, and click on the run button.
The output is plain text like the following:
Now let's also leverage the visualization. The next query gets all the software created by people known to "marko".
Copy the following query, paste it in the query input, and click on the run button.
The output is as follows. There are two paths in the result as "marko" knows "josh" who created "lop" and "ripple".
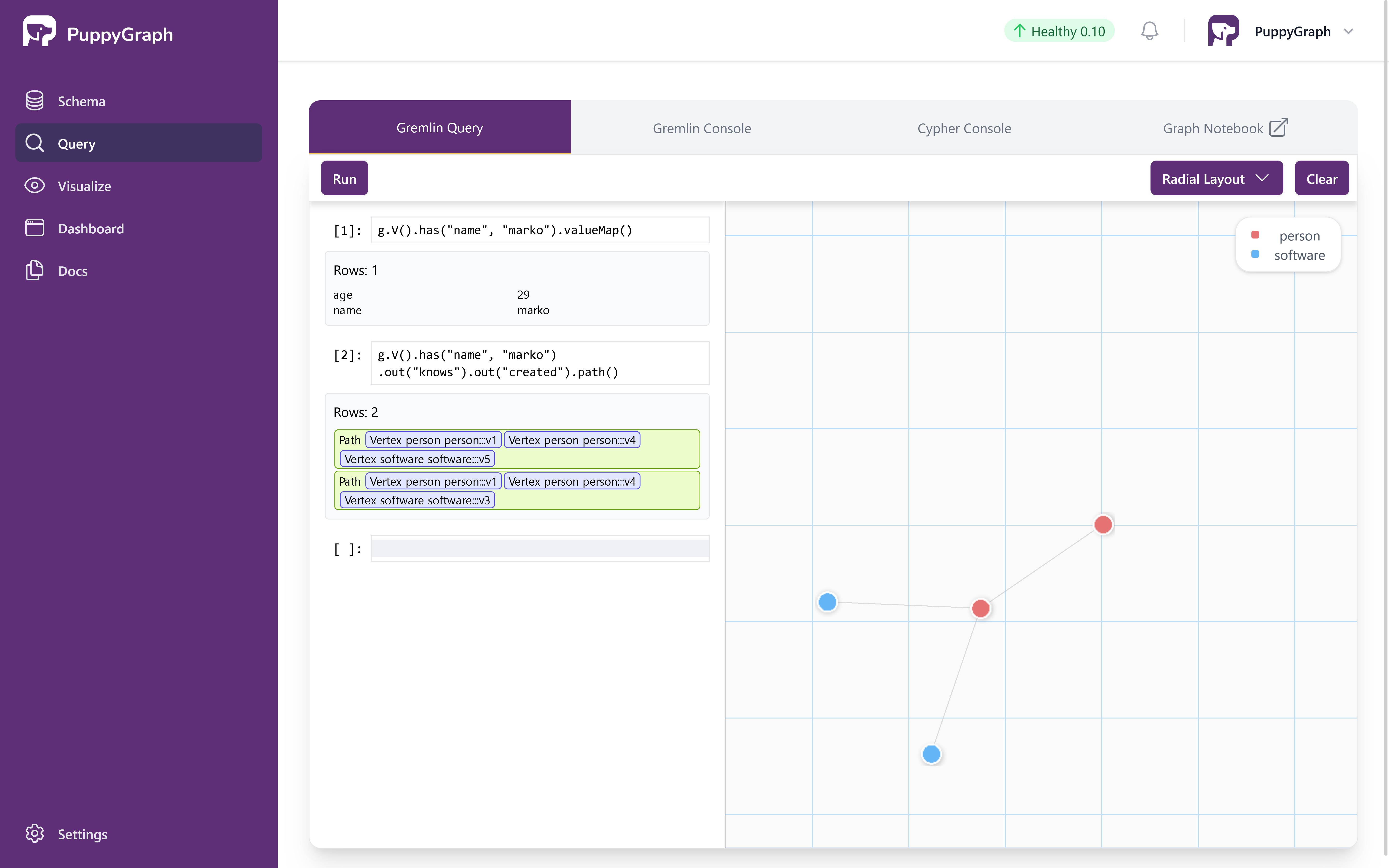
Interactive Query with Results
Alternative: Querying the graph via CLI
Alternatively, we can query the graph via CLI.
Execute the following command to access the PuppyGraph Gremlin CLI
The welcome screen appears as follows:
____ ____ _
| _ \ _ _ _ __ _ __ _ _ / ___| _ __ __ _ _ __ | |__
| |_) | | | | | | '_ \ | '_ \ | | | | | | _ | '__| / _` | | '_ \ | '_ \
| __/ | |_| | | |_) | | |_) | | |_| | | |_| | | | | (_| | | |_) | | | | |
|_| \__,_| | .__/ | .__/ \__, | \____| |_| \__,_| | .__/ |_| |_|
|_| |_| |___/ |_|
Welcome to PuppyGraph!
version: 0.10
puppy-gremlin>
Run the following queries in the console to query the Graph.
Properties of the person named "marko":
To exit PuppyGraph Gremlin Console, enter the command:
Cleaning up
Run the following command to shut down and remove the services: